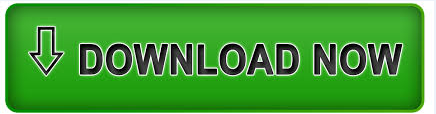
Based on the statistical results of correlation distribution in correlation map, we find that the positions of the pixel to be predicted and the most relevant pixel in reference block are not matched, and their correlation is inversely relevant to their distance. Specifically, we randomly select a point in the block to be coded, and then we calculate the correlation between this point and all pixels in reference blocks.
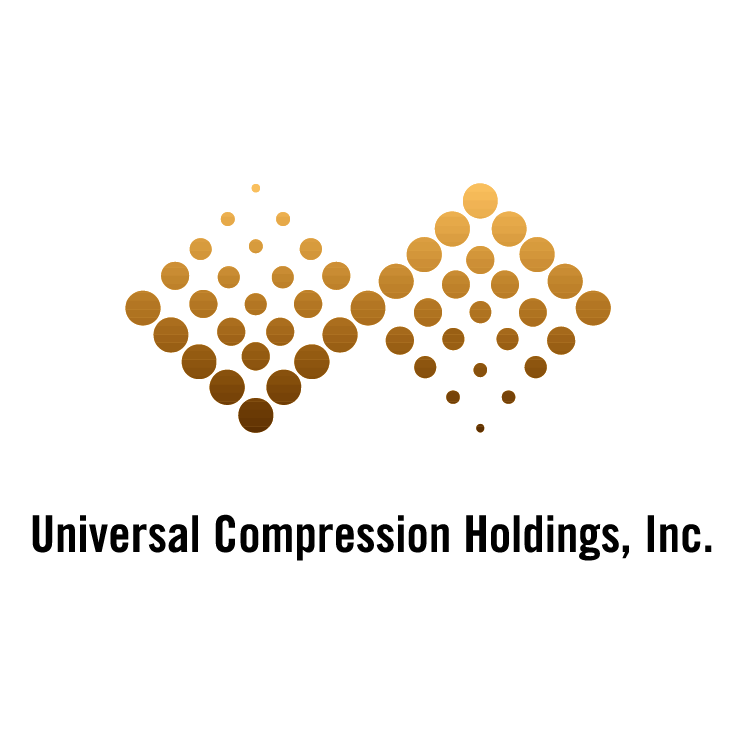
AI IMAGE COMPRESSION SOFTWARE FULL
READ FULL TEXT VIEW PDFįor better prediction among adjacent blocks, we analyze the correlation map between the point in predicted block and reference blocks, as shown in Figure 1. Metrics and promises obvious time-saving. State-of-the-art image compression methods in terms of both PSNR and MS-SSIM Post-processing module (BPM) with the importance of edge taken into account.Įxtensive experiments demonstrate that the proposed LBHIC codec outperforms Moreover, to alleviate blocking artifacts, we further propose a boundary-aware Pooling to extract the most relevant information in neighboring latent space. Is designed to better capture long-range correlations by utilizing the strip Neighbor pixels in traditional codecs, our contextual prediction module (CPM) Compared to prediction through linear weighting of Specifically, we introduceīlock partition and explicit learned predictive coding into learned imageĬompression framework. Win-win between coding performance and efficiency. Therefore, in order toĬollect their good qualities and offset their weakness, we explore a learnedīlock-based hybrid image compression (LBHIC) framework, which achieves a Compared to learned image codecs, traditional hybridĬoding frameworks are in general hand-crafted and lack the adaptability ofīeing optimized according to heterogeneous metrics. In a full-resolution manner thus not supporting acceleration via parallelismĪnd explicit prediction. Different from traditional hybrid coding frameworks, that areĬommonly block-based, existing learned image codecs usually process the images
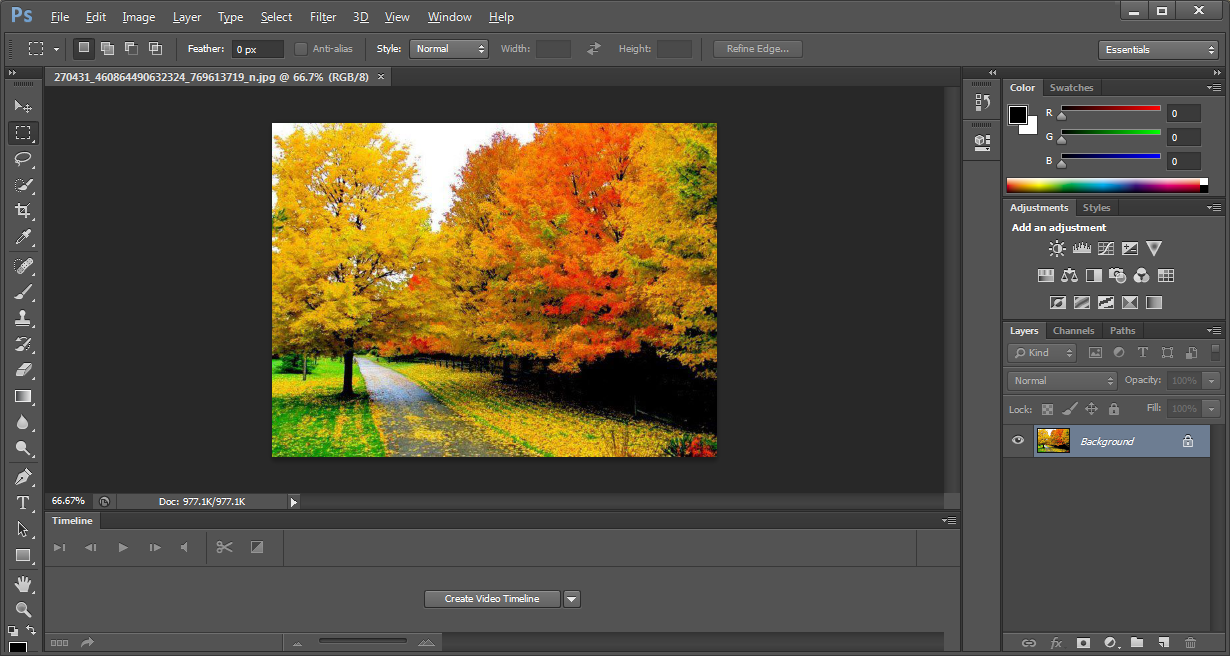
Thanks to its superiority in learning better representation through non- linear
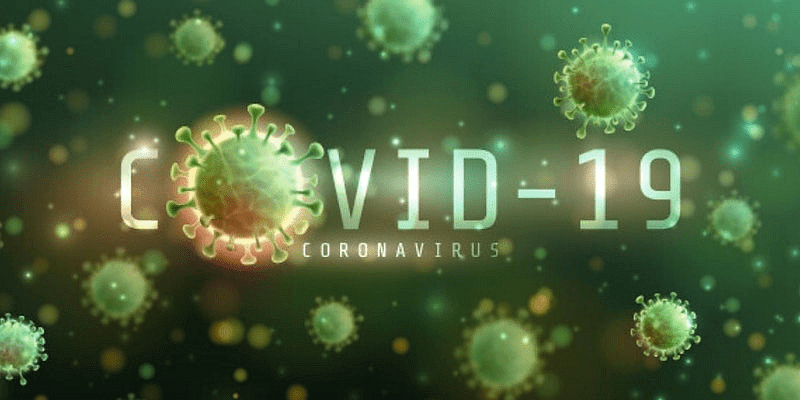
Learned image compression based on neural networks have made huge progress
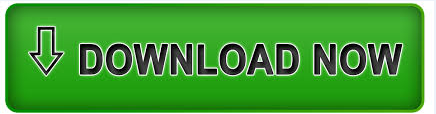